- ReTV Solutions: Supporting the Reuse of Broadcaster Collections Online - April 14, 2021
- 4u2 Messenger: Personalising the Distribution of Television Archives - February 24, 2021
- Reinvent & Adapt: TV in the Times of COVID-19 - April 9, 2020
Content personalisation is one of the key trends that continues to shape the media sector and form audience habits. Social media and content platforms are battling amongst each other to find the perfect recipe for a recommendation algorithm that would maximise the time users spend scrolling and binging content. But how can broadcasters and media archives use such content personalisation technologies to build long-lasting relationships with audiences?
4u2 Messenger
For this reason, ReTV developed the 4u2 Messenger application that automatically distributes video content from the media archive via messaging applications such as Facebook Messenger or Telegram. Unlike the already commonly used social media channels that address large audience segments, a messaging application allows us to reach individual users and tailor the video offering specifically to them. It pushes archival content into your information stream and invites users to provide immediate explicit feedback on videos via emoji reactions to gather input for further recommendations.
We automated the repetitive, time-consuming tasks of retrieving and curating videos and sending out messages on a large scale. With minimal editorial intervention, the Messenger curates and distributes video content based on its relevance at a particular moment in time for each individual user. Over time it also learns the optimal time to send out the videos for each individual user based on their past behaviour.
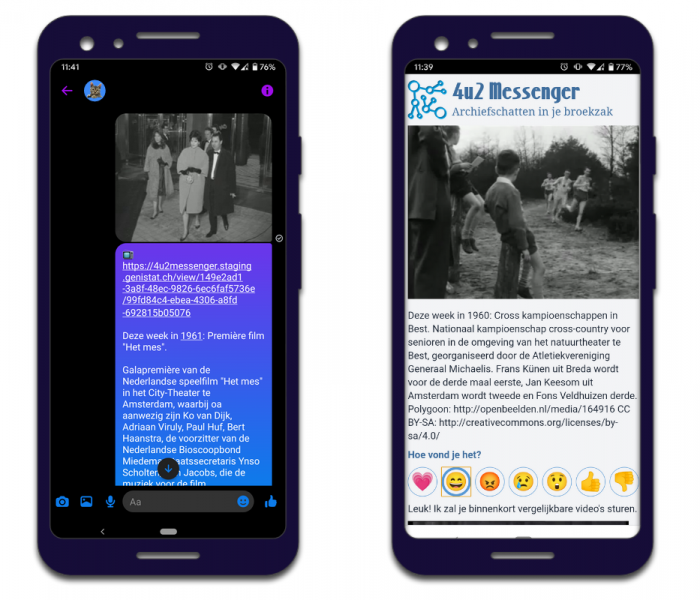
Recommendation Engine
The 4u2 Messenger was built with the specific goal in mind: to leverage the breadth of audiovisual archival content, especially the long tail of large-scale collections, in order to build long-lasting engagement between content owners and their audiences. It aims to strike a balance between the highly personalised and curiosity-inspiring approach, and to encourage exploration rather than mindless consumption.
The recommendation engine tries to satisfy the diverse and niche interests of its users with highly related content, but it also introduces a level of serendipity by recommending more unexpected videos. For example, a user who shows high preference for sports-related content would be regularly nudged to watch videos about topics that might have some overlap with sports. User reaction to this content would help to expand their bubbles of interest and create a more diverse profile.
Based on our initial evaluation with users, this element of surprise in the Messenger should keep audiences engaged for longer and spark their curiosity to discover new things. Most importantly, serendipity in the recommendation engine helps to avoid the danger of creating information bubbles that present only one side of the story and separate audiences into polarised camps that fail to see each other’s perspective.
The surfacing of video content from a large-scale collection is further supported by the use of ReTV’s Text-to-Video search to determine the relevance of videos. Instead of relying solely on video titles and descriptions in the metadata, the recommendation engine uses the visual analysis of each video represented via a vector to match user profiles with relevant content. This goes a long way to boost the visibility of videos that rarely get discovered due to their short or generic textual descriptions.
This Week in History
During the project, we customised the 4u2 Messenger specifically for the distribution of the Polygoon newsreel collection managed by the Netherlands Institute for Sound and Vision. These videos, dating between 1912 and 1949, need to be contextualised and presented in a new light to appeal to contemporary audiences.
We built the Messenger around the “This Week in History” concept popularised on social media platforms. The recommendation engine looks at the videos published in the same week across the years and uses this pool of videos to curate 2-3 videos per week for each user. The process of narrowing down the collection to individual weeks leaves between 15 and 30 videos to choose from and contributes to a more meaningful automated curation process given the seasonality of topics represented in the collection (for example, holidays or historic events commemorated annually). After watching the video, users can react with an emoji, and based on their reaction, the recommendation algorithm is adjusted to better match their interests.
Comments are closed.