- Summarizing videos on the Web - April 16, 2020
- ReTV award-winning paper at the MMM2020 - January 14, 2020
- The SUM-GAN-sl method for video summarization - October 22, 2019
THE ERA OF DIGITAL MEDIA
You wake up and check the weather forecast, your emails and social media notifications on your smartphone. You will likely be checking social media numerous times throughout the day. You view news content across various online channels. After work, you will watch videos, talk shows and TV series on a tablet at home. While browsing the web you will receive digital advertisements tailored to your preferences. This is typical user behavior that shows the dramatic impact of digital media on everyday life. Users, knowing what the technology can offer nowadays, spent less and less time on non-digital media. 21st century online audiences want to easily find content that is tailored to their personal preferences.
Technology is changing everything. The shift from traditional media to digital media is one to be noticed, understood and taken advantage of. TV content is also part of this shift. The rapidly changing digital media landscape continuously redefines the demands and expectations of TV viewers.
Yet, the very cause of this shift (hint: it is technology!) can also be the solution in turning this change into a new opportunity. To remain competitive in this new digital realm, broadcasters, broadcaster archives, and their content partners must embrace technology to adapt their content to this kind of online media consumption practices and find new routes to effectively reach the right audiences. They need to evolve into multi-channel content publishers, managing their traditional broadcasts on the Web, Smart TV and mobile apps, online video platforms, and social media and provide online access to archival material.
In this article, we talk about artificial intelligence technologies that have a direct application to media management, namely video temporal segmentation, video annotation with concepts and object labels, and automatic video summarization. Don’t worry, we won’t dive deep or use too much jargon.
DESCRIBING THE COMMON BUILDING BLOCKS
Video temporal segmentation
Videos can roughly be considered as collections of images, i.e., of the frames. However, each frame is usually not that different from the one preceding it, or the one following it. And, the fine differences from one frame to the other are critical pieces of information for understanding what each part of a video shows, e.g. that “a car is slowly moving on the street”. So, for the purpose of understanding what a video shows, the first analysis step is most often the identification of the video’s temporal structure.
As a first step, such methods detect the elementary building blocks of a video. These are called shots and are defined as sequences of frames captured uninterruptedly with the use of a single camera. Then, building on the extracted knowledge about the shot-level structure of the video they identify the story-telling parts of the video. These parts are called scenes and are formed by grouping consecutive shots into bigger sets, thus defining a less fine-grained segmentation of the video compared to shots.
However, when dealing with shots that have dynamic and gradually changing visual content, the shot-level segmentation can be too coarse and often fails to reveal useful information about their structure. Some recent and more sophisticated video temporal segmentation methods further decompose shots into more fine-grained and visually coherent parts, called sub-shots, that relate to different actions taking place during the video recording.
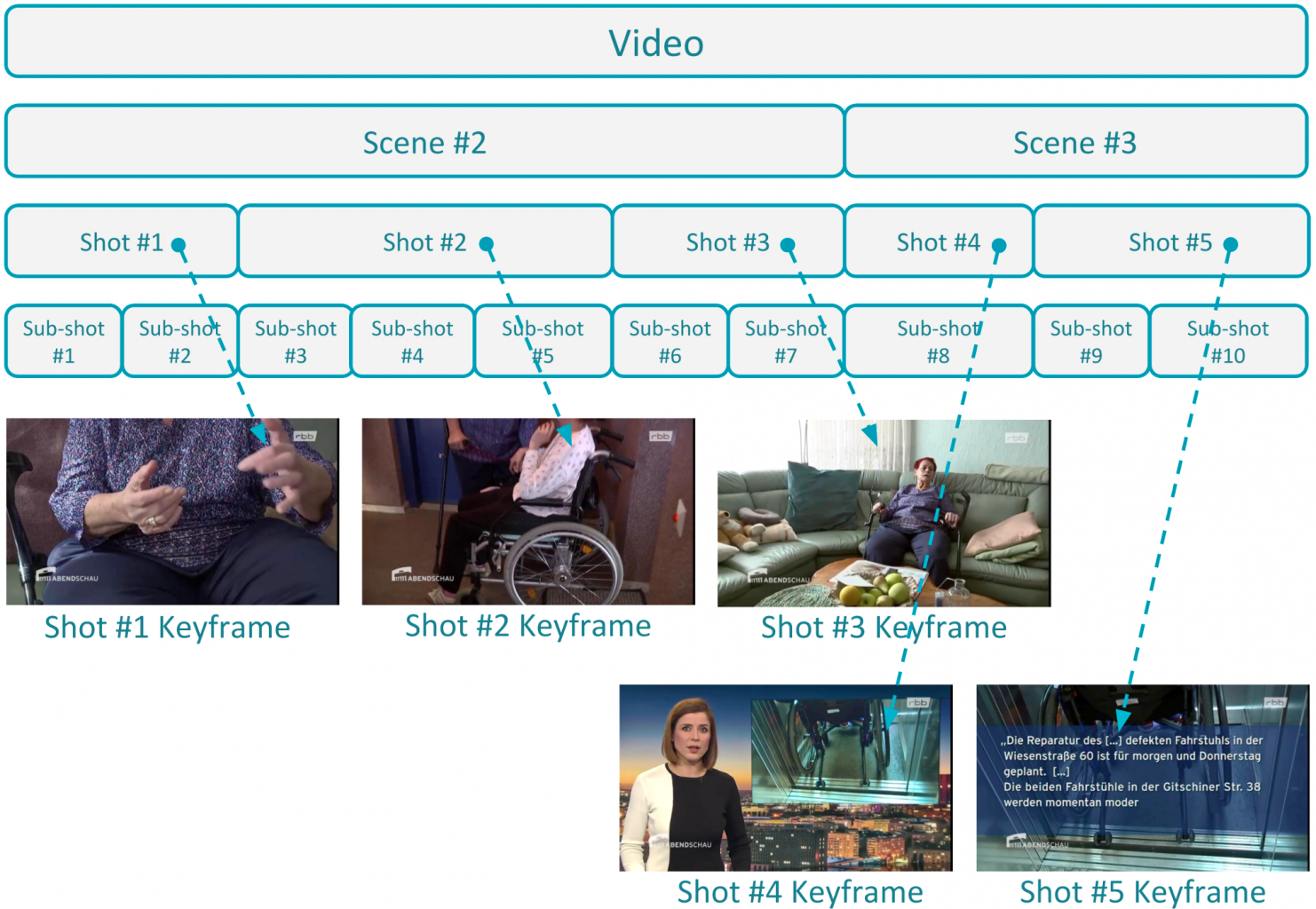
Concept Detection
They say “A picture is worth a thousand words”. This is because a human can easily describe the content of an image and extract useful knowledge. However, this is not the case for a machine. Computer scientists have to find a way for the machines to translate digital content data (literally zeros and ones) to semantic information. This is not an easy task, e.g., when the viewing conditions are different or the viewpoint is changed, this will lead to a significant change in the visual content. Yet, “a car driving down the highway” – an example of a semantic description for a short video clip – has the same meaning despite the car being blue or red, no matter the location of the highway, no matter the time of the day.
Concept detection is the automatic extraction of high-level concepts by using low-level features, i.e. to annotate an image with one or more labels describing its semantic content using some pre-defined concepts. Concepts may refer to objects (e.g., “car” and “chair”), activities (e.g., “running” and “dancing”), scenes (e.g., “hills” and “beach”).
In recent years there have been many large-scale image classification and concept detection competitions in the scientific community. Keeping with our promise to not use too much jargon, we just state that there is a common trend to use deep convolutional neural networks, a framework inspired from the way the human brain works. Utilizing these sophisticated structures computer scientists have achieved a breakthrough in the performance and accuracy of concept detection methods. This means, in the digital TV world, that you can now know where exactly in the video a, e.g., “police chase” can be seen, without someone manually adding such annotations to the different video parts.
Object Detection
Object detection is a group of methods that deal with detecting and localizing instances of semantic objects of a certain class (such as humans, buildings, or cars) in images or videos. It is a field that attracted a lot of attention in commercial, as well as research, applications. The application of object detection spans from surveillance to real-time vehicle detection in smart cities and pedestrian detection. Taking into account the long-term interest of the scientific community in such applications, we can say that the field of object detection is relatively mature. Additionally, thanks to advances in modern hardware, breakthroughs in this field have been ground-breaking. Object detection can help self-driving cars safely navigate through traffic, detect violent behavior in a crowded place, ensures proper quality control of parts in manufacturing, among many other things. In digital TV applications, object detection can highlight the region of a video frame where a product logo is found, thus, easily infer when and where a specific brand is advertised.
Automatic video summarization
The aforementioned AI techniques can be further combined to perform even higher-level (in the conceptual sense) tasks, for example, to automatically summarize a video. The main idea of summarization is to find a subset of data that contains the “information” of the entire set, i.e., in our case, the shortening of a video, in order to create a summary that still conveys the major points of the original contents.
This is a challenging problem because, among others, humans would summarize a video based on their subjective criteria; thus, it is difficult to define a clear and objective set of rules for automating this task. However, once again due to years of research and borrowing knowledge from different subfields of AI (computer vision, unsupervised learning techniques such as clustering, learning-based methods, recurrent neural networks, oops too much jargon!), promising solutions have started to emerge.
Combining the blocks
We will illustrate a few examples where AI (specifically the aforementioned methods) can practically help broadcasters, broadcaster archives and their content partners in the age of digital media.
The results of video temporal segmentation analysis, the defined video scenes, shots, and sub-shots, serve as input to the rest of AI algorithms. Moreover, the extracted information about the temporal structure of the video can build an annotation model by defining the segments of each video in a corpus.
The detected concepts can then be used for the purpose of automatically annotating the videos with a human-interpretable semantic description. First and foremost concept detection is the basic step to extend the annotation model with automatically derived metadata. This can further enable semantic indexing and ease the retrieval of videos based on concept-based queries, or extract knowledge from the viewing patterns of the user.
Combining the power of object detection with video temporal segmentation, we can detect the channel logo of TV stations or recognize brand logos in TV programs. We can automatically label TV content segments as advertisements, additionally providing the metadata about the subject of the advertisement. Consequently, we can exclude advertisements from videos or target different advertisements to specific users.
A smart video summarization system can help the process of automatically generating thematic video summaries. Besides this, it can be customizable. It can be content-oriented, e.g., sports highlights detection. It can be user-oriented, i.e., different for each target group of users by taking advantage of the explicitly stated user preferences or relying on information inferred from their viewing patterns. It can also be adapted according to the target vector’s characteristics, e.g., produce summaries of an optimal duration for each vector (here, the term “vectors” refers to the different means for distributing the content. e.g. Smart TV, Web, and mobile applications, various social media platforms). Furthermore, different types of summaries can be generated such as video teasers, i.e, giving out a few details about a given video and certainly not giving away any plot twists! Finally, a smart summarization system cannot ignore the human factor in the whole process: it must offer the user the functionality to easily edit/refine the automatically generated video summaries and automate tedious tasks such as sharing them on multiple social media platforms.
THE RETV PROJECT
ReTV brings together computer scientists, broadcasters, interactive TV companies, audio-visual archives from across Europe for innovation in digital media. Combining the technological know-how, and state-of-the-art implementations of the aforementioned AI techniques with the insight and the knowledge of professionals in the broadcasting area, we want to demonstrate how advanced technological solutions can effectively manage different vectors, optimize and promote content to broader audiences, automate the planning and publication of content and iteratively monitor and enhance this workflow, thus unlocking the full potential in digital media environments. The novel services that ReTV offers will ultimately help broadcasters to stay competitive in the digital TV environment in this age of digital media.
Author: Kostas Apostolidis and Vasileios Mezaris, CERTH-ITI
Comments are closed.