- Artificial Intelligence in the Audiovisual Industry - April 29, 2020
- Content Wizard: Editorial support as the new frontier of content distribution - February 18, 2020
- Content sWitch for a modern content experience - May 21, 2019
Forecasting the audience for a particular TV show is an old, but still relevant problem. It is important to know the future audience of a show as precisely as possible to optimise the buying of ads. If an ad-break underperforms, the TV channel has to compensate the advertiser with additional placements for free that make up the difference. On the other hand, if an ad-break overperforms, the TV channel has sold it too cheaply.
Audience forecasts could be run for different scenarios, and be employed to test different scheduling strategies in advance. A TV channel could, for example, compare the forecasts for a schedule that has multiple small ad-breaks with a schedule where there is one large ad-break.
Basic forecasting based on past audience sizes usually works fine for homogeneous, repetitive content such as TV series. Obviously, there can still be some systematic trends, like seasonal fluctuations or an audience losing the interest in a particular show, but this can be predicted with traditional time series models as long as the changes don’t happen too quickly.
However, what we cannot easily forecast with time series models are special, one-time events that affect the consumption of TV content, like big sports events or breaking news. See for example the audience graph for the German national broadcaster Das Erste on Zattoo. The big peak to the right is the final of the German football cup.
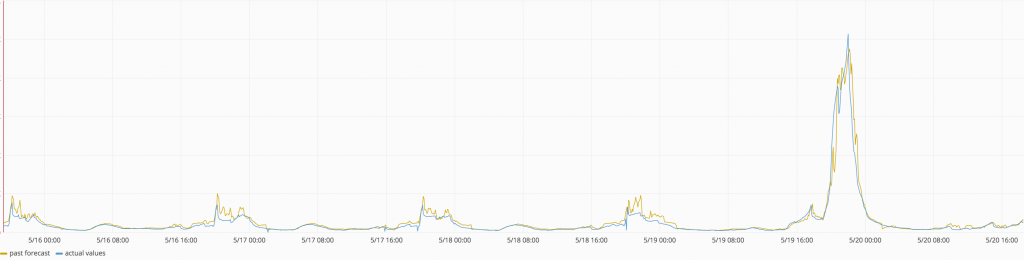
Seven days of predicted and actual audience for German national broadcaster on Zattoo.
Our predictions using a time series model were surprisingly good since the event obviously does not follow the weekly seasonality. A Saturday evening football game is still a relatively common event and easier to predict than the Australian Open tennis final on a Friday morning: In Switzerland for example, the audience numbers are significantly higher if either Roger Federer or Stan Wawrinka play in a tennis match. Their participation is however often not clear until one or two days before, in which case the TV channels will reschedule their program accordingly. The graph also nicely shows that getting the special events right is important because they attract very large audiences.
More robust TV audience forecasting requires the smart combination of various sources of data, structured and unstructured:
- TV audiences metrics, including viewing interests & behavioral patterns, spatiotemporal & socio-demographic information
- social media & other textual reactions to TV-broadcasted events such as Tweets, Likes, and reviews
- attributes extracted from video and audio: which person says what, which celebrity is present, what is visible in the picture (e.g. detect nudity and gore).
A prerequisite is Electronic Program Guide (EPG) information which is accurate to the second because this tells us with what TV content users are interacting & engaging. This is never the case at present, as can easily be seen by the fact that this data does not contain information on ads: When one program ends the next one is assumed to start immediately. Again, it is especially important that this information is correct for sports events or other special, one-time shows, which usually do not conform to the long-term TV schedule.
Tennis matches, for example, have no predefined length. They might take a lot longer or end sooner than originally planned in the TV-program. If the EPG data is not retrospectively updated, the audience numbers are assigned to the wrong content. For instance, it has happened in the past, that the Simpsons episode scheduled after a tennis match had the highest rating of the day. The game lasted longer than planned, and the tennis audience, therefore, spilled into the Simpsons episode. This required manually correcting the EPG information in hindsight.
Audience forecasts are a crucial component of the ReTV project. ReTV is aiming to repurpose TV content across multiple vectors (TV Channel website, video apps, social media etc.) and maximise viewership engagement while doing so. This means that the correct piece of content needs to be published on the vector that has the highest forecasted viewer engagement. Classical TV audience forecasting was for a static piece of content (a TV show) and one vector (linear TV). ReTV is now going further, by building forecasting models that predict the audience for different versions of a TV show (e.g. long summary vs. short summary) across multiple vectors. To achieve this ReTV is working on the following:
- video & textual transcripts fragmentation & annotation
- matching TV content to structured knowledge bases on external events
- video adaptation and summarization
- optimized scheduling across publication vectors
TV content is often segmented into different topics. An obvious example is the evening news that discusses multiple, often separate topics in sequence. Understanding TV content, not only on a show level but on the much finer level of individual content segments or scenes, will allow for automatically personalised content.
Together with our future ability to predict the impact of a particular, newly generated piece of content will allow ReTV to offer truly personalised TV in an automatic fashion.
Authors: Krzysztof Ciesielski and Basil Philipp from Genistat.
Comments are closed.